Author: Mona Sobhani, PhD
UPDATE: see the full post on Medium here and my conversation with Charlie Warzel of the New York Times Privacy Project here.
I’ve been thinking for a while now that the definition of Personal Health Information (PHI) is outdated and I’ll explain why below. Because of this fact, one of two things are true. Either the federal law that protects PHI, HIPAA: (1) should apply to all types of personal data, including location, activity, and social), or (2) is no longer relevant, and broader personal data regulations are needed to encompass all data related to health.
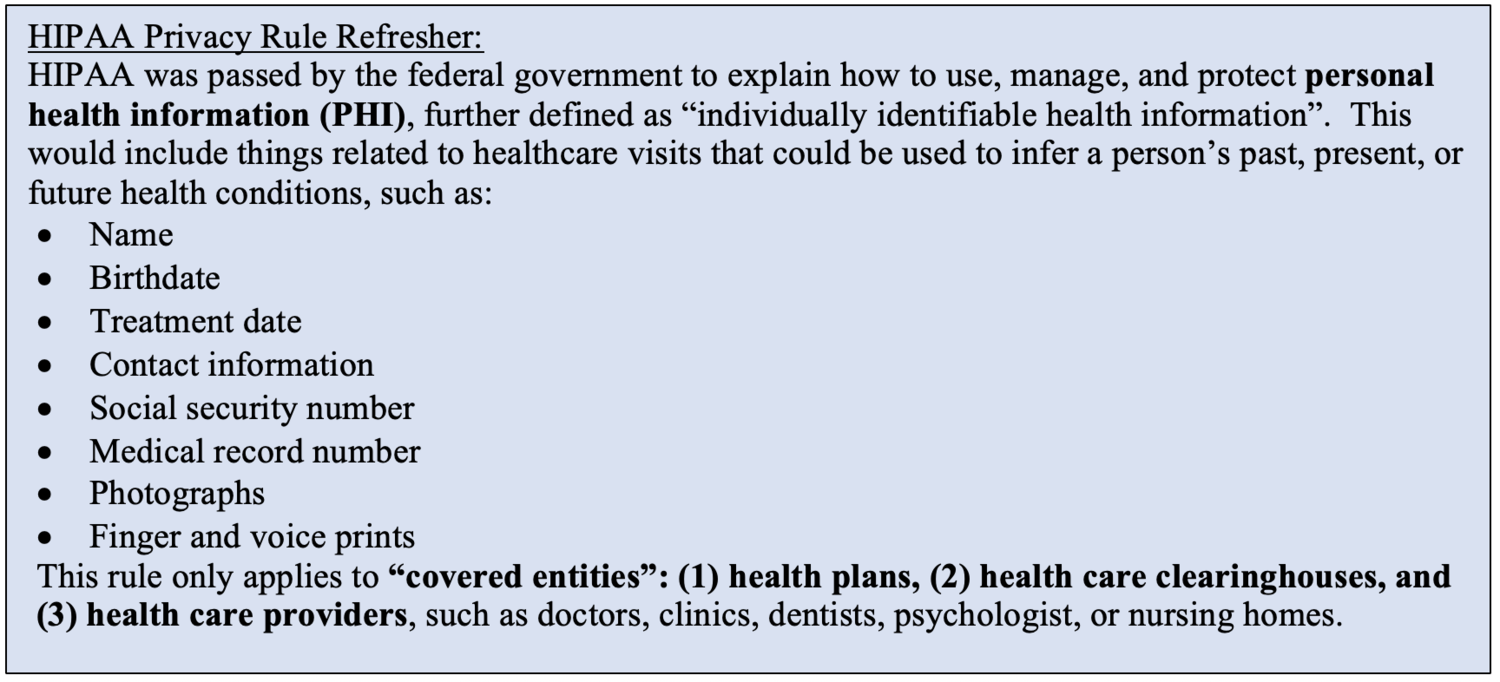
To be clear, HIPAA is a wonderful legislation that protects patients. Without HIPAA, a health plan could sell a patient’s data without the patient’s permission to, let’s say, an employer who could use it for company decisions, or to a bank who could use the information to deny a loan. The problem with HIPAA is that it makes one huge assumption: that health status can only be inferred by the types of personal health data covered by HIPAA.
In this era, though, can we confidently say which personal data is related to health and which is not? Social determinants of health, such as poverty, education, gender, ethnicity, and employment account for ~60-80% of our health (1–3) – and all those types of information can be collected, or at least inferred, by the massive amounts of personal information that is now readily collected online. Beyond social determinants of health, specific social network data and physical activity, sleep, and social interaction data correlates with, and can even predict, depression, suicide risk, and mental illness (4–8), as well as risky behavior that can lead to bad health outcomes (9). The predictions are so strong that Facebook has a suicide prediction and intervention program (10). If all of this personal data can help predict past, current and future health states, shouldn’t it also be protected?
Some may argue that, yes, with all this de-identified, aggregated data, we can predict health trends, but it’s all anonymous, so what’s the issue? In fact, many companies and organizations have claimed that sharing de-identified data is not a privacy risk. However, we now know that it can be rather easy to re-identify many different types of data. For example, a recent study showed over 90% accuracy of re-identifying individuals from physical activity data and demographic data (11). Re-identification has also been found to be possible using online search data (12), movie rating data (13) , social network data (14), genetic data (15), social network metadata (16), and wearable data (17). A recent, and terrifying, New York Times article (18) shows how location data can be used to identify individuals, because it turns out there’s only one person who lives where you do, works where you do, and that has your exact daily routine.
Even if the definition of PHI were to change to include these other data, tech companies would probably still not be considered “covered entities” – even though they are the largest hoarders of our personal data. With the current digital landscape, either HIPAA needs to be updated to cover all relevant data and entities, or maybe we need new regulatory frameworks.
REFERENCES
1. O’Neill Hayes T, Delk R. Understanding the Social Determinants of Health.; 2018. https://www.americanactionforum.org/research/understanding-the-social-determinants-of-health/#_edn9.
2. Racial and Ethnic Health Disparities What State Legislators Need to Know.; 2013. http://myhealthoutcomes. Accessed January 25, 2019.
3. Magnan S. Social Determinants of Health 101 for Health Care: Five Plus Five. NAM Perspect. 2017;7(10). doi:10.31478/201710c.
4. Eichstaedt JC, Smith RJ, Merchant RM, et al. Facebook language predicts depression in medical records. Proc Natl Acad Sci. 2018;115(44):11203-11208. doi:10.1073/PNAS.1802331115.
5. Wang R, Chen F, Chen Z, et al. StudentLife. In: Proceedings of the 2014 ACM International Joint Conference on Pervasive and Ubiquitous Computing – UbiComp ’14 Adjunct. New York, New York, USA: ACM Press; 2014:3-14. doi:10.1145/2632048.2632054.
6. Rabbi M, Ali S, Choudhury T, Berke E. Passive and In-situ Assessment of Mental and Physical Well-being using Mobile Sensors. Proc . ACM Int Conf Ubiquitous Comput UbiComp. 2011;2011:385-394. doi:10.1145/2030112.2030164.
7. Puiatti A, Mudda S, Giordano S, Mayora O. Smartphone-centred wearable sensors network for monitoring patients with bipolar disorder. In: 2011 Annual International Conference of the IEEE Engineering in Medicine and Biology Society. IEEE; 2011:3644-3647. doi:10.1109/IEMBS.2011.6090613.
8. De Choudhury M, Gamon M, Counts S, Horvitz E. Predicting Depression via Social Media. http://course.duruofei.com/wp-content/uploads/2015/05/Choudhury_Predicting-Depression-via-Social-Media_ICWSM13.pdf. Accessed June 13, 2017.
9. Rivers C, Lewis B, Young S. Detecting the Determinants of Health in Social Media. 2012. doi:10.1371/journal.pcbi.1002616.
10. Singer N. In Screening for Suicide Risk, Facebook Takes on Tricky Public Health Role. The New York Times. https://www.nytimes.com/2018/12/31/technology/facebook-suicide-screening-algorithm.html?mc_cid=03a239a9bd&mc_eid=105181941b. Published December 31, 2018.
11. Na L, Yang C, Lo C-C, Zhao F, Fukuoka Y, Aswani A. Feasibility of Reidentifying Individuals in Large National Physical Activity Data Sets From Which Protected Health Information Has Been Removed With Use of Machine Learning. JAMA Netw Open. 2018;1(8):e186040. doi:10.1001/jamanetworkopen.2018.6040.
12. Barbaro M, Zeller T, Hansell S. A Face is Exposed for aol searcher no. 4417749. The New York Times. https://www.nytimes.com/2006/08/09/technology/09aol.html?mtrref=www.google.com&gwh=82E5F9FB0A49332F37DFB3048879099B&gwt=pay. Published August 9, 2006.
13. Narayanan A, Shmatikov V. Robust De-anonymization of Large Sparse Datasets. In: 2008 IEEE Symposium on Security and Privacy (Sp 2008). IEEE; 2008:111-125. doi:10.1109/SP.2008.33.
14. Narayanan A, Shmatikov V. De-anonymizing Social Networks. In: 2009 30th IEEE Symposium on Security and Privacy. IEEE; 2009:173-187. doi:10.1109/SP.2009.22.
15. Gymrek M, McGuire AL, Golan D, Halperin E, Erlich Y. Identifying Personal Genomes by Surname Inference. Science (80- ). 2013;339(6117):321-324. doi:10.1126/science.1125339.
16. Perez B, Musolesi M, Stringhini G. You Are Your Metadata: Identification and Obfuscation of Social Media Users Using Metadata Information.; 2018. www.aaai.org. Accessed August 15, 2018.
17. Lane ND, Xie J, Moscibroda T, Zhao F. On the feasibility of user de-anonymization from shared mobile sensor data. In: Proceedings of the Third International Workshop on Sensing Applications on Mobile Phones – PhoneSense ’12. New York, New York, USA: ACM Press; 2012:1-5. doi:10.1145/2389148.2389151.
18. Valentino-DeVries J, Singer N, Keller MH, Krolik A. Your Apps Know Where You Were Last Night, and They’re Not Keeping It Secret. The New York Times. https://www.nytimes.com/interactive/2018/12/10/business/location-data-privacy-apps.html. Published December 10, 2018.